Jake Yeung
Analytical Methods and Computational Biology
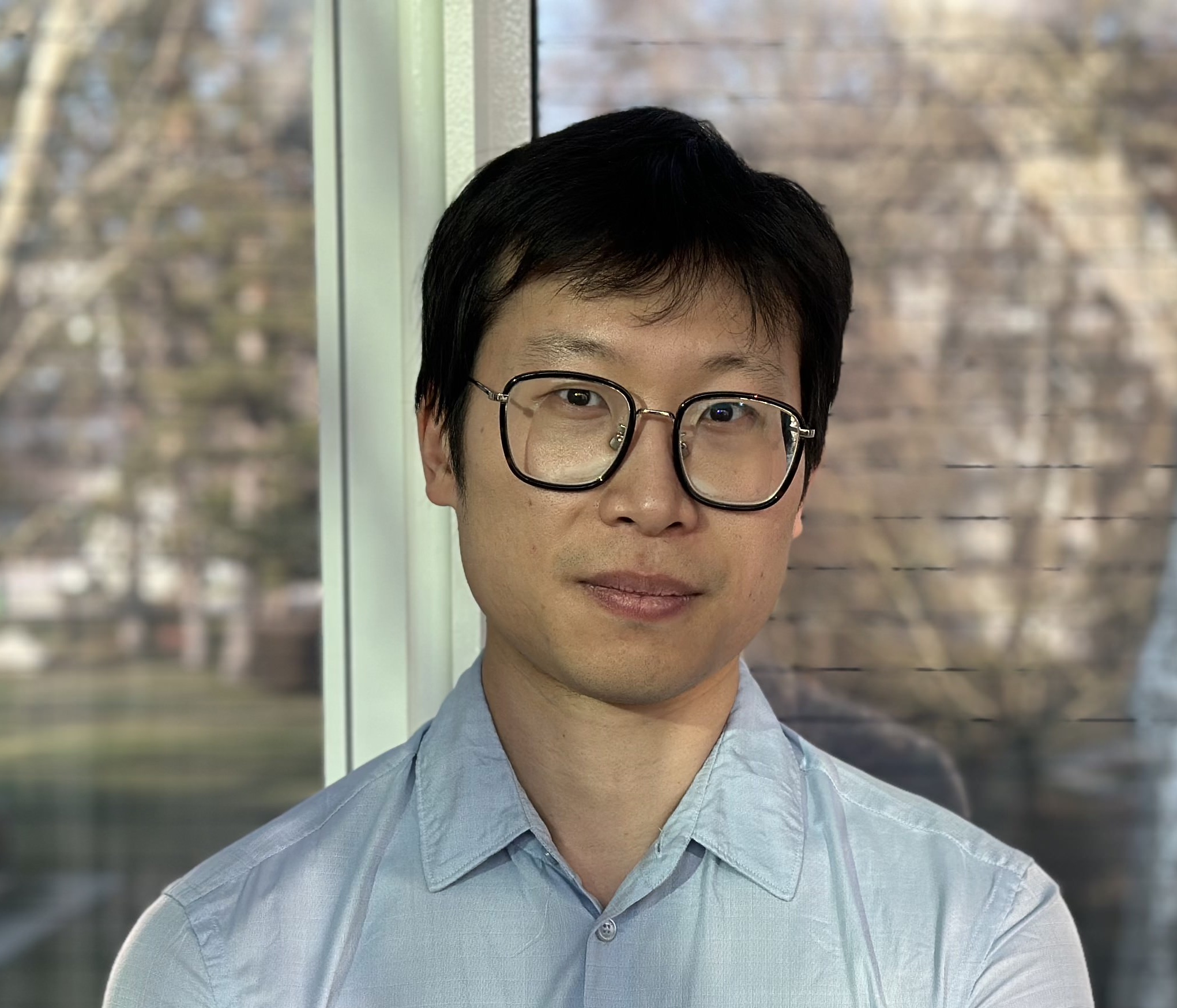
Principal Scientist.
Genentech, Inc.
1 DNA Way,
South San Francisco, CA 94080, USA
Overview
I am a computational biologist working at the interface of statistical/machine learning, functional genomics technologies, and genome biology. I focus on understanding how different cells turn/off different parts of our genome to give rise to different functions in our body, and how this control fails in disease.
For my training, I was a Human Frontier Science Program (HFSP) fellow with Alexander van Oudenaarden at the Hubrecht Institute. Before that, I completed my PhD at the EPFL in computational systems biology advised by Felix Naef.
Research themes
My projects span three approaches: computational methods, technology development, and biological function. They often pairs two of these approaches at a time (Fig. 1), bringing in wet and dry lab components.
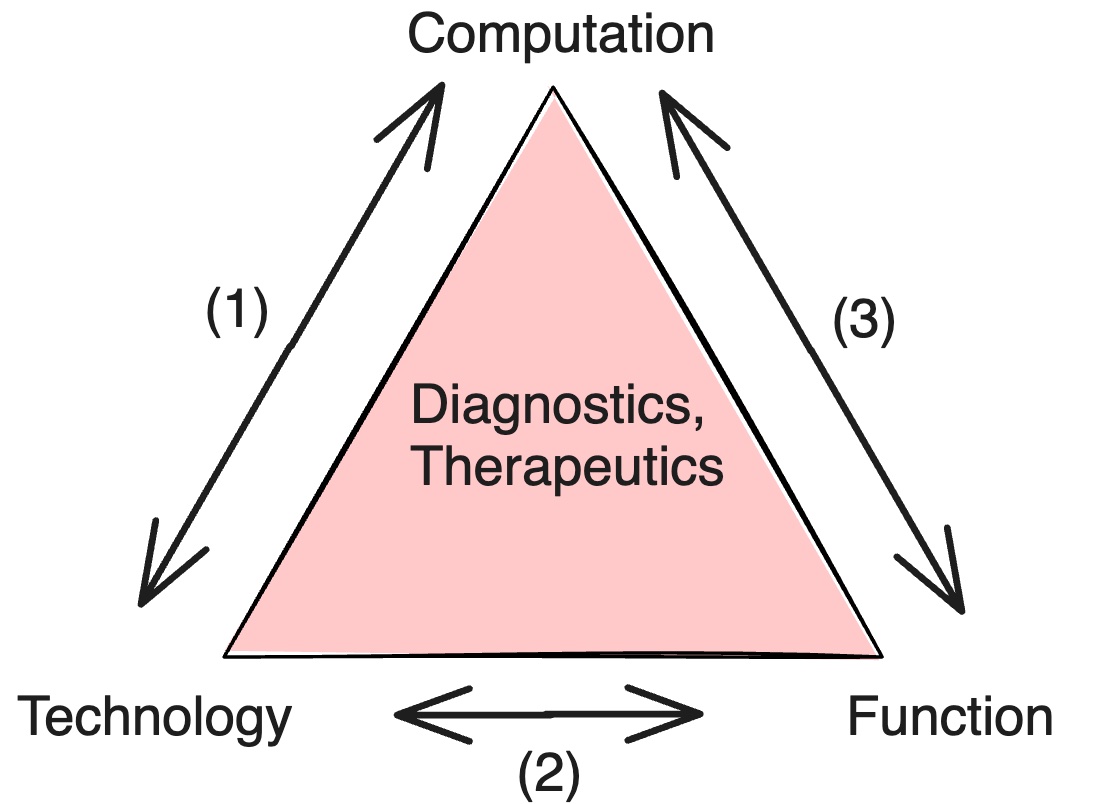
(1) Computationally-driven experimental design
Computation ↔technology development.
Inferring new information from single-cell experiments allow us to ask new questions about how cells function. Many wet lab-driven approaches have advanced what we kinds of biological measurements we can make, but trying to experimentally enumerate all possible combinations of measurements or conditions can quickly hit diminishing returns. Developing new statistical methods alongside with new technologies can advance measurement limitations by inferring hidden biological parameters or unmixing multiplexed experiments.
Recently, we have recently developed a statistical unmixing method (scChIX-seq) that maps multiple histone modifications in single cells and learns the correlation structure between histone marks (Yeung*, Florescu*, Zeller* et al 2023). We are building on these multiplexing/unmixing methods to perform large-scale genetic and drug screening.
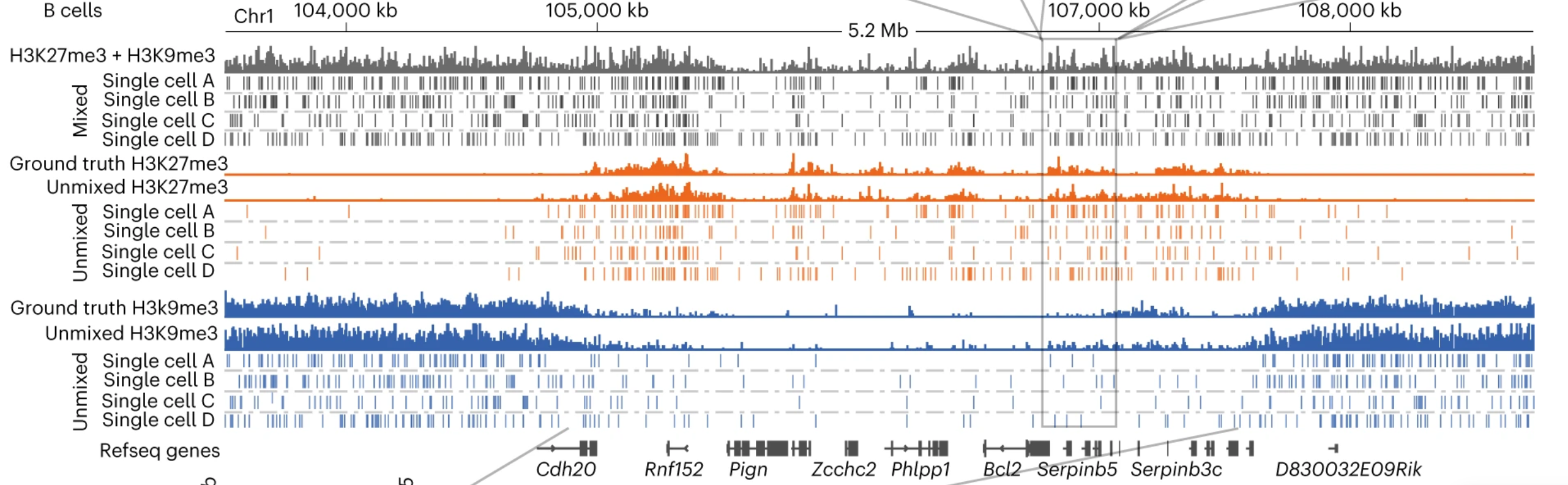
(2) Principles of data analysis for new functional genomics technologies
Tech dev ↔ biological function.
New functional genomics technologies can now measure the regulation and output of every gene in the genome in virtually every cell in the body. However, the analysis side is challenging because it is unclear (a) what are the most exciting biological questions you can ask from different technolgoies given a fixed budget, (b) how to think about the design and analysis to separate biological signal from unwanted technical artifacts in sparse, noisy, and limited data regimes, and (c) how to integrate external information from other experiments across different labs in the world (where conditions vastly differ) to improve statistical inference and prediction.
Recently, we have shown that combining cell surface markers with histone modification mapping in single cells (sortChIC) can systematically compare the genome-wide dfferences between active (e.g. H3K4me1, H3K4me3) and repressed (e.g. H3K27me3, H3K9me3) chromatin states during blood maturation(Zeller*, Yeung* et al 2022). We are now developing new ways to infer time information and integrate that in the analysis.
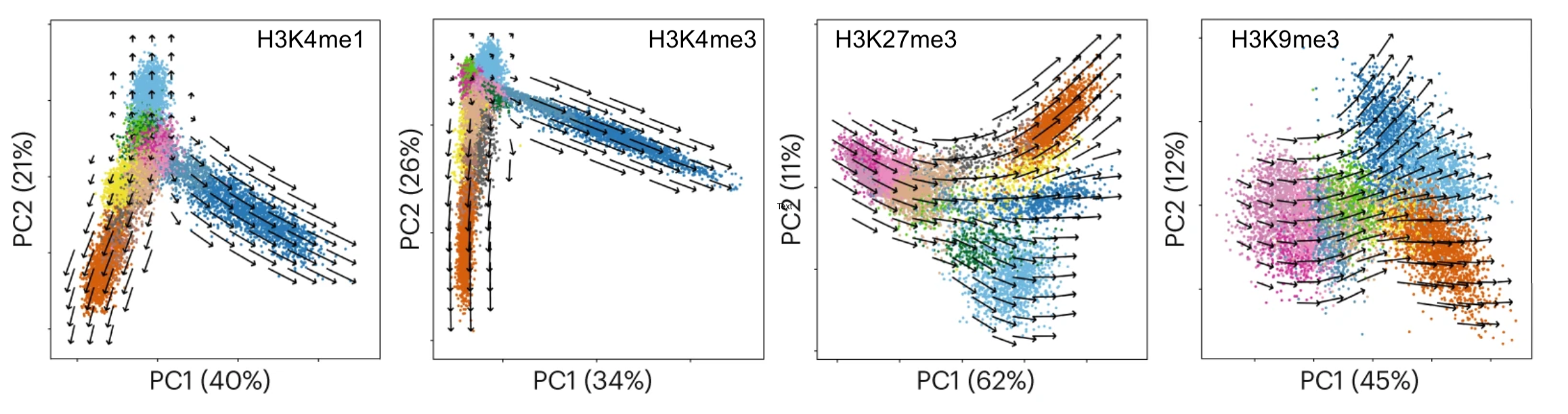
(3) Asking how cells regulate their genomes
Computation ↔ biological function.
Although functional genomics technologies can now measure the expression and accessibility of thousands of genes across thousands of cells, discovering regulatory principles that explain the changes in gene expression over time and in different cell types is still challenging. Even when using established technologies, the analysis side remains the bottleneck because one must still redefine/refine what are the interesting questions to ask given a specific experiment, and craft the analysis to systematically tackle the question that will inspire the next incisive experiment. Possible principles are hypothesized through data exploration, then articulated in a statistical model, and tested against carefully crafted null hypotheses. At each step one must reason about the outputs in the context of how and why the experiment was performed.
Recently, we have crafted model selection approaches to systematically categorize genes into a handful of groups that share similar regulation. These methods allow the diverse gene expression patterns in a biological tissue to be explained in terms of a small number of biological regulatory principles, for example quantifying how much tissue-specific dynamics come from individual transcription factors versus cooperation between pairs (Yeung*, Mermet* et al 2018) or the contribution of sleep-wake driven versus circadian processes in gene expression dynamics (Hor*, Yeung* et al 2019). These analyses lead to direct predictions that can be experimentally tested (for example, CRISPR knockout of enhancers Mermet*, Yeung* et al 2018).
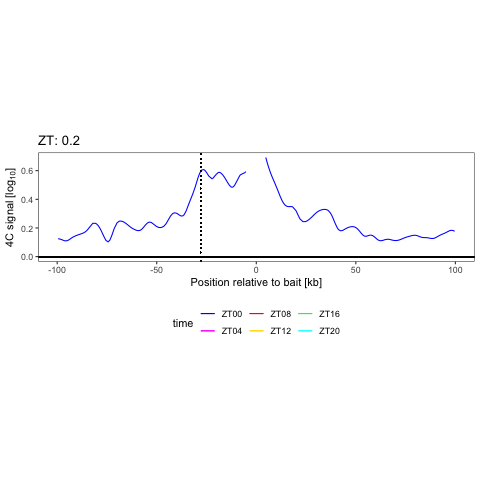